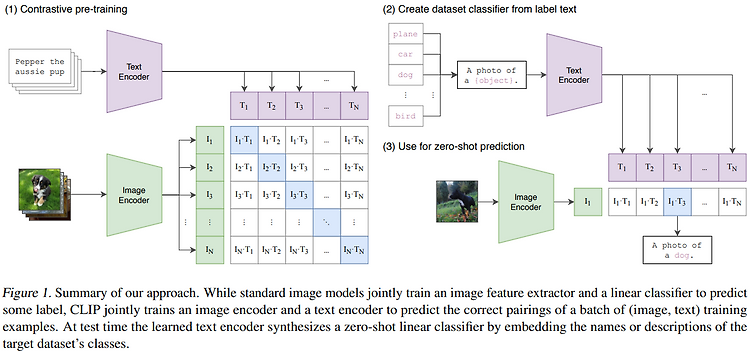
[๋
ผ๋ฌธ ๋ฆฌ๋ทฐ] Learning Transferable Visual Models From Natural Language Supervision / CLIP / Multi-modal network
ยท
๐ Research/Multi-modal
Open AI์์ ๊ฒ์ฌํ(ICML2021) Contrastive Language-Image Pre-training(CLIP)๋ฅผ ์ ์ํ ๋
ผ๋ฌธ์ ์๊ฐํฉ๋๋ค. Introduction & Motivation ๋ฅ๋ฌ๋์ด computer vision์ ๊ฑฐ์ ๋ชจ๋ ๋ถ์ผ์์ ๊ต์ฅํ ์ ํ์ฉ๋์ง๋ง ํ์ฌ ์ ๊ทผ ๋ฐฉ์์๋ ๋ช๊ฐ์ง ๋ฌธ์ ๊ฐ ์์ต๋๋ค. ๊ธฐ์กด์ vision model๋ค์ ํ์ต๋ task์๋ ์ฑ๋ฅ์ด ์ฐ์ํ์ง๋ง ์๋ก์ด task์ ์ ์ฉ์ํค๊ธฐ ์ํด์๋ ์๋ก ํ์ต์ ์ํค์ผ ํ๋(๊ทธ๋ฌ๋ฉด ์๋ก์ด ๋ฐ์ดํฐ์
๊ณผ ์ถ๊ฐ ๋ ์ด๋ธ๋ง์ด ํ์..) ๋ฒ๊ฑฐ๋ก์(?) ์ด ์์ต๋๋ค. ๋ฒค์น๋งํฌ์์ ์ ์ํ๋๋ ๋ช๋ช model๋ค์ stress test์์ ์ข์ง ์์ ๊ฒฐ๊ณผ๋ฅผ ๋ณด์ฌ์ฃผ๊ธฐ๋ ํฉ๋๋ค. ๋์์ผ๋ก raw text์ image๋ฅผ pair๋ก ํ์ต์ํค๋ ๋ฐฉ๋ฒ..